
Operationalizing AI is about bridging the gap between research and reality, embedding intelligence in everyday tools to solve pressing challenges effectively.
Artificial intelligence (AI) has the potential to revolutionize business operations by automating tasks, improving decision-making, and driving innovation. However, deploying AI is not a one-time project but an ongoing process that requires careful planning, execution, and maintenance.
AI Operationalization
AI has become a key driver of innovation and transformation in various industries, and businesses are increasingly seeking ways to leverage its potential for competitive advantage. However, deploying AI in real-world scenarios requires a structured approach to ensure its successful operationalization.
AI operationalization refers to the process of putting AI into operation or production, including the preparation, development, deployment, and ongoing management of AI systems.
It involves several steps, each with its unique challenges and considerations. The first step is to understand the business problem and identify use cases for AI implementation.
Once the use cases are identified, businesses need to develop an AI strategy that outlines the approach to operationalizing AI, including the selection of appropriate AI models, data preparation, and model deployment.
Developing an AI strategy requires a comprehensive understanding of the business objectives, the available data, and the resources required to execute the strategy.
It also entails identifying the key stakeholders, including customers, employees, and partners, and understanding their expectations and concerns about AI implementation.
The next step in AI operationalization is data preparation, which involves the collection, cleaning, preprocessing, and labeling of data to ensure its relevance and quality for AI models. The quality of data is critical in determining the accuracy and effectiveness of AI models in real-world scenarios.
Once data is prepared, businesses need to select and develop appropriate AI models, including machine learning algorithms, deep learning models, and others, depending on the nature of the business problem and the available data.
Model development involves training, testing, and validating the models to ensure their accuracy and reliability.
After selecting and developing AI models, businesses need to deploy them into production environments, ensuring scalability, reliability, and security. This involves integrating AI models into existing business systems, monitoring their performance, and ensuring continuous improvement.

Understanding AI Operationalization Challenges
AI operationalization presents several challenges that businesses need to address to ensure successful implementation. One of the main challenges is the availability of high-quality and relevant data for AI models. Data must be representative of the real-world scenarios and should be unbiased and of sufficient volume to support the AI models’ accuracy.
Another challenge is the shortage of skilled AI professionals who can develop and deploy AI models. Businesses need to invest in talent acquisition and development and build a culture of continuous learning to address this challenge.
Regulatory compliance is another critical challenge in AI operationalization, as businesses need to adhere to various regulations regarding data privacy, security, and ethical AI use. Failure to comply with these regulations can result in legal and reputational consequences.
Finally, change management presents a significant challenge in AI operationalization, as businesses need to ensure that employees and stakeholders are onboard with AI implementation and understand its potential benefits for the business.
Operationalizing AI requires a structured approach that involves several steps, including understanding the business problem, developing an AI strategy, data preparation, model selection and development, model deployment, and ongoing management.
However, several challenges need to be addressed to ensure successful AI implementation, including data quality, talent shortage, regulatory compliance, and change management.
Developing an AI Strategy
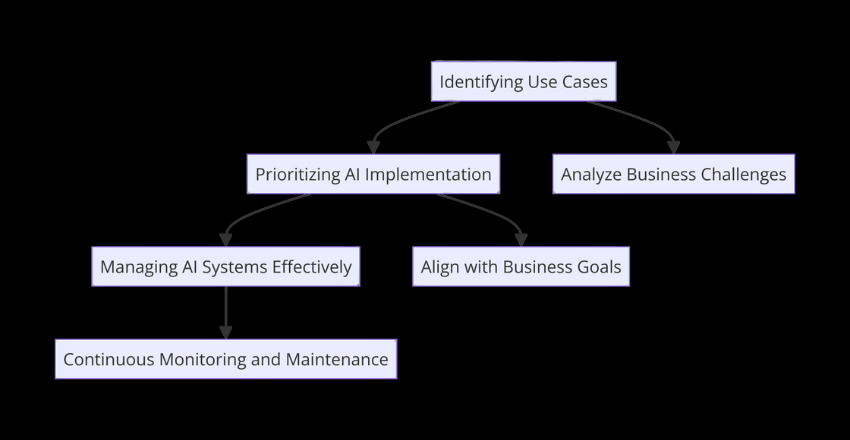
Successfully operationalizing AI requires businesses to have a comprehensive strategy that outlines how AI will be integrated into their operations. Developing an AI strategy involves identifying use cases, prioritizing AI implementation, and managing AI systems effectively. An effective AI strategy must be flexible enough to adapt to changes in business operations and technology advancements.
Identifying Use Cases
The first step in developing an AI strategy is identifying use cases where AI can be effectively used to transform business processes. Businesses must evaluate various business operations, workflows, and data sources to identify areas where AI can be deployed to drive innovation and efficiency.
Identifying use cases requires a thorough analysis of business challenges and opportunities, and selecting the most promising ones to focus on.
Prioritizing AI Implementation
Once potential use cases have been identified, businesses must prioritize which use cases to implement first. Prioritization should be based on factors such as potential impact, level of difficulty, and feasibility. It is important to ensure that the chosen use cases are aligned with the organization’s overall business goals.
Prioritization enables businesses to realize outcomes quickly while building momentum for further AI implementation.
Managing AI Systems Effectively
The success of AI implementation depends largely on how effectively businesses manage their AI systems. AI systems require continuous monitoring, maintenance, and improvement to ensure they function optimally. This involves developing processes for system management, incorporating updates, and maintaining data quality.
Creating an AI operations team responsible for managing AI systems can help ensure that the systems are operating efficiently, and issues are quickly identified and addressed.
Overall, developing an AI strategy requires businesses to identify how AI can be effectively used to transform business processes, prioritize implementation, and manage AI systems effectively. Doing so can help businesses achieve their goals while leveraging the power of AI to improve their operations.
Data Preparation for AI
One of the most critical steps in operationalizing AI is data preparation. It involves collecting, cleaning, and transforming raw data into a format suitable for AI model training and testing. High-quality and relevant data is essential to ensure the accuracy and effectiveness of AI models.
AI data preparation involves several techniques such as data preprocessing, which includes data normalization, feature scaling, and handling missing data. It is also important to clean the data by removing duplicates, outliers, and irrelevant entries.
Data governance plays a crucial role in AI operationalization as it ensures that the data used for training and inference is reliable, accurate, and compliant with data privacy regulations. Therefore, it is vital to establish data governance policies and practices to maintain the quality and integrity of data used for AI.
Data Preprocessing for AI
Data preprocessing is a crucial step in data preparation, which involves transforming raw data into a format suitable for AI model training and testing. Data preprocessing techniques include data normalization, scaling, and dimensionality reduction.
Data normalization is the process of scaling numerical data to fall within a specific range or distribution. Feature scaling is another technique used to normalize data by bringing different features to the same scale.
Handling missing data is another critical aspect of data preprocessing. Missing data can lead to inaccurate AI models, and therefore, it is important to fill the missing values or remove them.
Data preparation is a fundamental step in the operationalization of AI. AI models depend on high-quality and relevant data, which requires careful preparation techniques such as preprocessing and data governance to ensure accuracy, integrity, and compliance with regulations.
AI Model Selection and Development

One of the critical steps in operationalizing AI is selecting the right AI model and developing algorithms that can effectively address business challenges. The initial phase involves identifying the business problem that requires an AI solution and understanding the available data.
This information can help determine the type of AI model required for the specific task at hand.
When selecting an AI model, it’s essential to consider factors like model complexity, accuracy, and interpretability. Complex models may deliver higher accuracy, but they may also be challenging to interpret and explain to stakeholders.
On the other hand, simple models can be easily interpreted, but they may not deliver the accuracy required for the specific business problem.
Once the AI model is selected, the next step is to develop and train the AI algorithms. This process includes defining the training data, selecting the hyperparameters, and optimizing the model for accuracy.
Developing an AI algorithm is an iterative process that requires continuous evaluation and refinement to ensure its effectiveness.
Various tools and resources are available for developing AI algorithms, ranging from open-source libraries to proprietary software. Businesses must select the tools that align with their specific needs and expertise. Additionally, having a skilled workforce capable of developing and improving AI algorithms is necessary for successful AI operationalization.

Continuous Model Improvement
AI model development doesn’t end with the successful deployment of an AI system. Continuous monitoring, evaluation, and improvement are essential to ensure the model’s effectiveness and relevance in the ever-evolving business environment.
By monitoring model performance and user feedback, businesses can identify areas for improvement and implement changes to continuously enhance the model’s accuracy and effectiveness.
AI model development is a crucial stage in the operationalization process, and businesses must invest the required resources and skills to ensure successful implementation.
AI Model Deployment
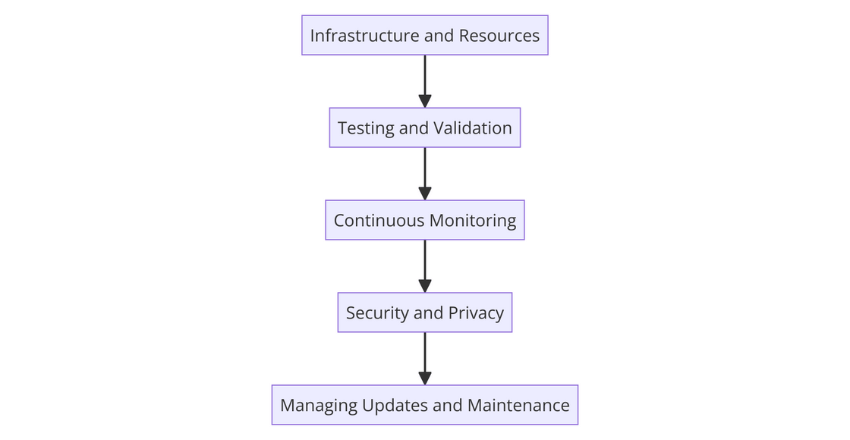
Deploying AI systems into production requires careful planning and execution to ensure scalability, reliability, and security. Here are some considerations and best practices for successful AI model deployment:
Infrastructure and Resources
Before deploying AI models, businesses need to ensure that the necessary infrastructure and resources are in place. This includes hardware, software, and network requirements, as well as storage and processing capabilities. Cloud-based solutions can be a viable option for businesses looking to minimize infrastructure costs and scale quickly.
Testing and Validation
Prior to deployment, AI models should be thoroughly tested and validated to ensure their accuracy and effectiveness. This involves evaluating the performance of the model on real-world data and verifying that it meets the desired outcome. It is also important to ensure that the model does not exhibit any biases or unintended consequences that could impact its performance in production.
Continuous Monitoring
Once deployed, AI models should be continuously monitored to ensure that they are performing as expected. This involves setting up monitoring tools and alerts to identify any issues or errors as soon as they occur. Regular performance evaluations and assessments should also be conducted to identify areas for improvement and optimization.
Security and Privacy

Deploying AI systems also requires attention to security and privacy considerations. This includes ensuring that data is protected and encrypted in transit and at rest, and implementing access controls to prevent unauthorized access. Businesses should also be aware of regulatory requirements and standards for data privacy and security, and ensure compliance with these regulations.
Managing Updates and Maintenance
AI models are not static and require regular updates and maintenance to ensure continued effectiveness. This includes updating the model with new data and features, and retraining the model as necessary. Businesses should establish processes for managing updates and maintenance, and assign responsibilities to the relevant teams and individuals.
Deploying AI systems presents unique challenges, but with careful planning and execution, businesses can successfully operationalize AI and reap the benefits of this transformative technology.
Performance Monitoring and Evaluation
One of the critical aspects of operationalizing AI is ongoing performance monitoring and evaluation. AI systems need to be continuously assessed to ensure they are delivering the desired outcomes and providing value to the business.
Effective AI performance monitoring involves defining relevant metrics that can be used to evaluate the effectiveness of the AI system. These metrics can include accuracy, precision, recall, and F1 score, among others. It is essential to establish a feedback loop that allows for continuous evaluation and improvement of the AI system, to ensure that it remains effective and relevant over time.
Evaluating the effectiveness of an AI system involves assessing how well it performs against defined metrics. This can involve conducting A/B testing, comparing the performance of the AI system with that of a human expert or other AI systems. It can also involve assessing the system’s ability to handle edge cases, exceptions, and unexpected inputs.
AI system evaluation is crucial in identifying performance bottlenecks, areas for improvement, and potential risks. It informs decisions about whether to scale up or scale down AI deployment, modify the AI model, or change the data sources used to train the AI system.
Ensuring Ethical AI Use
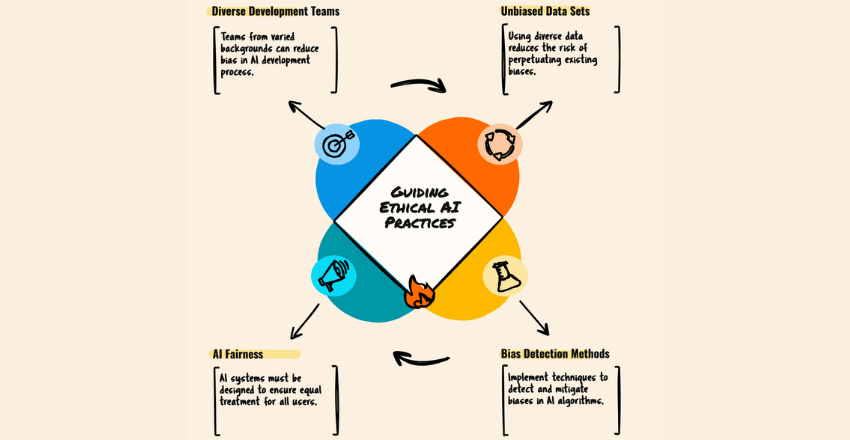
As artificial intelligence becomes more prevalent in business operations, it is crucial to ensure its ethical use to avoid negative consequences. AI systems can perpetuate societal biases and discrimination if not developed and deployed ethically.
Ethical AI use involves mitigating AI biases, ensuring AI fairness, and maintaining transparency in AI systems. One of the ways to mitigate biases in AI systems is by ensuring that diverse teams develop and test AI models using unbiased datasets. It is also necessary to employ technical techniques like data preprocessing and bias detection to minimize biases in AI models.
AI fairness is another critical aspect of ethical AI use. An AI system should treat all individuals equally, regardless of their race, religion, gender, or any other defining characteristic. Ethical AI use involves ensuring that AI systems do not perpetuate implicit bias or discrimination.
Transparency in AI systems is crucial to maintain trust between stakeholders. AI systems should provide clear explanations of how decisions are made, the data used in decision-making, and any other relevant information. Businesses that ensure ethical AI use build trust with stakeholders and promote confidence in their operations.
Managing AI Operations
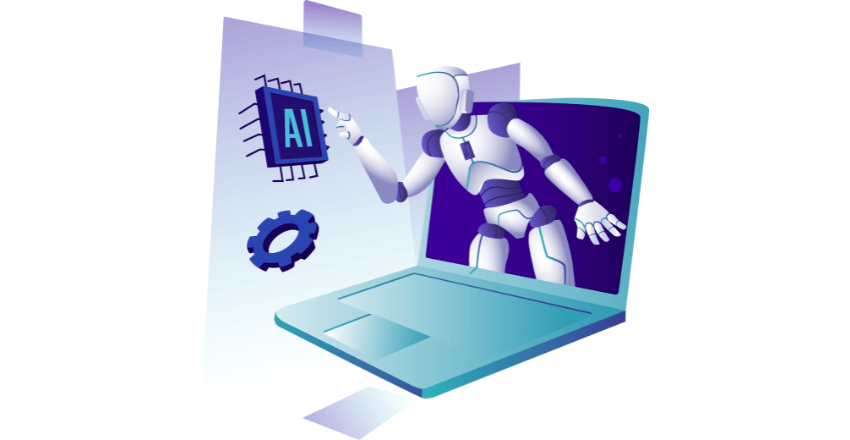
Operationalizing AI is an ongoing process that requires effective management to ensure smooth functioning of AI systems. This includes managing AI operations, system maintenance, incident response, and resource allocation.
Effective AI operations management involves establishing clear processes and procedures for managing AI systems in production. This includes managing system performance, ensuring scalability, reliability, and security, and optimizing resource utilization.
System maintenance is a critical aspect of ensuring the continued smooth functioning of AI systems. This includes monitoring system health, identifying and resolving issues, and performing regular maintenance tasks such as updates, backups, and security checks.
Incident response is crucial for promptly addressing issues that arise during AI operations. This includes establishing incident response procedures, identifying the root cause of the issue, and implementing preventive measures to mitigate the risk of future incidents.
Effective management of AI operations requires having a dedicated AI operations team with the required expertise and skillset. This includes having AI engineers, data scientists, and AI operations specialists who can work together to ensure that AI systems operate smoothly and efficiently.
Managing AI operations involves effective management, system maintenance, incident response, and resource allocation to ensure smooth functioning of AI systems. It requires having a dedicated team with the necessary expertise and skillset to manage AI systems effectively.
Overcoming Challenges in Operationalizing AI
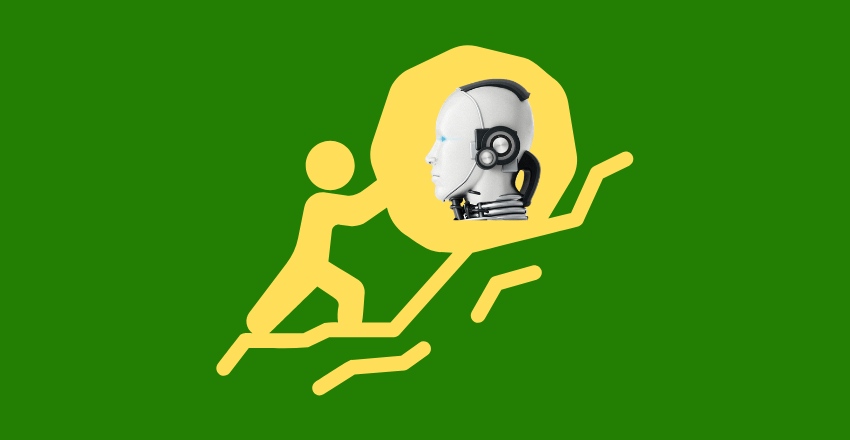
While the potential benefits of operationalizing AI are vast, businesses face significant challenges in implementing and scaling AI systems. From data quality to talent shortages, the hurdles to successful AI implementation are numerous.
Data Quality
Data quality remains a critical challenge in operationalizing AI. Poor data quality leads to inaccurate and biased AI models, which can negatively impact business operations and outcomes. To overcome this challenge, businesses need to invest in data governance and management strategies, ensuring that data is accurate, relevant, and consistent.
Talent Shortages
The shortage of skilled AI talent is a major obstacle to AI implementation, as businesses require a diverse skill set to operationalize AI successfully. While some businesses opt for outsourcing AI talent, others invest in upskilling their existing workforce. Building a comprehensive AI team requires a combination of data scientists, machine learning experts, and domain experts.
Regulatory Compliance
Regulatory compliance is an increasingly important consideration for businesses operationalizing AI. With the rapid growth of AI adoption, new regulations and guidelines are emerging, and businesses must ensure that their AI systems comply with these regulations. Building ethical and transparent AI systems that comply with data privacy laws is crucial for building trust with stakeholders.
Change Management
Operationalizing AI requires significant changes to business processes, culture, and mindset. Resistance to change can derail AI implementation efforts, leading to a lack of adoption and underutilization of AI systems. To overcome this challenge, businesses need to develop effective change management strategies, ensuring that stakeholders are engaged and prepared for the changes that AI will bring.
Resource Constraints
Implementing and scaling AI systems requires significant resource investments, including hardware, software, and infrastructure. Businesses must allocate resources effectively, ensuring that AI systems are developed and deployed efficiently. From cloud-based AI platforms to automation tools, businesses must invest in the right resources to realize the benefits of AI.
Overcoming the challenges of operationalizing AI requires a comprehensive strategy that addresses the technical, organizational, and cultural aspects of AI adoption.
By developing a clear AI strategy, investing in data quality and governance, building diverse AI teams, ensuring regulatory compliance, managing change effectively, and allocating resources efficiently, businesses can overcome the hurdles to successful AI implementation and reap the rewards of a transformed digital landscape.
AI Governance and Compliance
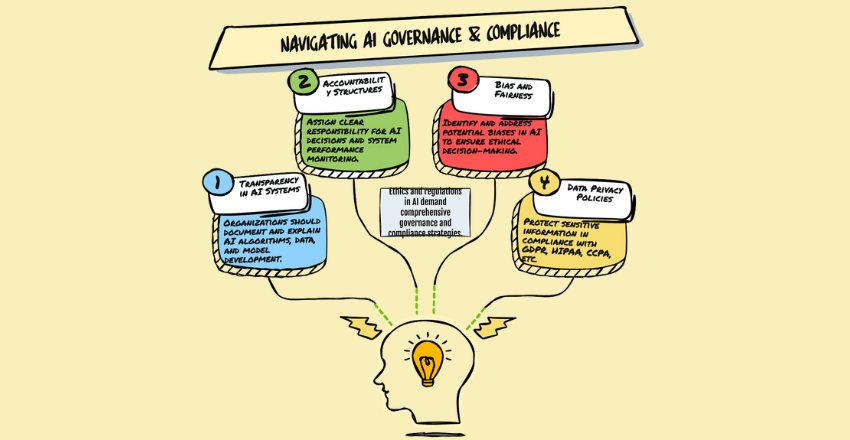
As businesses increasingly incorporate AI into their operations, it is crucial to ensure that ethical principles are upheld and regulatory requirements are met. This requires the establishment of robust AI governance and compliance frameworks.
A key aspect of AI governance is the need for transparency and accountability. Organizations must be able to explain how their AI systems work and the decisions they make. This includes documenting AI algorithms, data sources, and the process of model development. It is also important to establish clear lines of responsibility for AI system performance and ensure that any potential biases are identified and mitigated.
AI compliance is another critical aspect of operationalizing AI. Organizations must comply with relevant regulations such as GDPR, HIPAA, and CCPA, depending on their geographic location and industry. It is important to establish data privacy policies and governance structures to ensure that sensitive data is protected and used ethically.
Regulatory requirements for AI are still evolving, making it essential for businesses to stay up-to-date with the latest developments. In some cases, businesses may need to conduct risk assessments and obtain regulatory approvals before deploying AI systems into production.
Overall, AI governance and compliance are crucial for building trust with customers, employees, and other stakeholders. By adopting responsible AI practices and complying with regulations, organizations can ensure that their AI systems are fair, transparent, and ethical.
Examples of Operationalized AI
Operationalizing AI has already shown significant success across various industries, improving business processes, and enhancing customer experiences. Below are a few examples of businesses that have successfully implemented AI:
1. Healthcare Industry:
One of the most significant impacts of AI in the healthcare industry is the ability to analyze large volumes of medical data quickly and accurately. For example, New York Genome Center uses IBM Watson’s AI capabilities to analyze patient records, symptoms, and medical histories to identify potential diseases or conditions. AI also enables personalized treatment plans, reducing recovery time and preventing hospital readmissions.
2. Retail Industry:
One of the most prominent use cases of AI in the retail industry is personalized product recommendations. Amazon uses AI algorithms to analyze customer behavior and purchase history to provide personalized recommendations, resulting in more significant sales and improved customer satisfaction.
3. Financial Services:
AI has revolutionized the financial industry, providing valuable insights into customer behavior, fraud detection, and risk management. JP Morgan Chase uses AI to analyze customer data and detect fraudulent transactions in real-time, reducing financial risk. Capital One uses AI chatbots to provide customer service, reducing wait times and enhancing the customer experience.
These examples show how AI can improve various aspects of business operations, resulting in increased efficiency, cost savings, and better customer experiences.
Wrapping up

Operationalizing AI is no longer a futuristic concept; it is a necessary step for businesses seeking to stay competitive in the digital age. Successfully operationalizing AI requires a comprehensive strategy, effective data preparation, AI model selection and development, performance monitoring, and ongoing management.
Despite the challenges businesses may face during the process, the benefits of AI operationalization are vast. Organizations that successfully operationalize AI can significantly improve efficiency, reduce costs, and drive innovation.
Operationalizing AI is a journey, and each step is critical. By understanding the process, challenges, and best practices discussed in this article, businesses can lay the foundation for successful AI deployment. The time for AI is now, and the potential is limitless.
External Resources
https://www.ibm.com/topics/ai-governance
FAQ

1. How do you prepare data for an AI model?
Answer: Preparing data is crucial for the success of AI models. It involves cleaning, normalization, and splitting into training and testing sets. Here’s a Python code sample using Pandas and Scikit-learn for data preparation:
import pandas as pd
from sklearn.model_selection import train_test_split
from sklearn.preprocessing import StandardScaler
# Load data
data = pd.read_csv('data.csv')
# Clean data
data.dropna(inplace=True) # Remove missing values
# Feature scaling
scaler = StandardScaler()
scaled_features = scaler.fit_transform(data.drop('target_column', axis=1))
# Split data
X_train, X_test, y_train, y_test = train_test_split(scaled_features, data['target_column'], test_size=0.2)
This code snippet demonstrates basic steps in data preparation: cleaning, scaling, and splitting, which are essential before training an AI model.
2. What’s involved in training an AI model?
Answer: Training an AI model involves selecting a suitable algorithm, feeding it data, and adjusting parameters to improve accuracy. Here’s an example using TensorFlow to train a simple neural network:
import tensorflow as tf
# Define the model
model = tf.keras.Sequential([
tf.keras.layers.Dense(10, activation='relu', input_shape=(X_train.shape[1],)),
tf.keras.layers.Dense(1, activation='sigmoid')
])
# Compile the model
model.compile(optimizer='adam', loss='binary_crossentropy', metrics=['accuracy'])
# Train the model
model.fit(X_train, y_train, epochs=10, validation_split=0.2)
This code creates a neural network for binary classification, compiles it with an optimizer and loss function, and trains it on the data.
3. How do you deploy an AI model for use in applications?
Answer: Deploying an AI model involves making it available for making predictions in a production environment. Here’s an example of saving a model with TensorFlow and loading it for inference:
# Save the trained model
model.save('my_model.h5')
# Load the model
loaded_model = tf.keras.models.load_model('my_model.h5')
# Use the model for predictions
predictions = loaded_model.predict(X_test)
This example shows how to save a trained model to a file and then load it for making predictions. Deployment might also involve setting up a web or cloud service to make the model accessible via API calls.

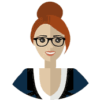
Jane Watson is a seasoned expert in AI development and a prominent author for the “Hire AI Developer” blog. With over a decade of experience in the field, Jane has established herself as a leading authority in AI app and website development, as well as AI backend integrations. Her expertise extends to managing dedicated development teams, including AI developers, Machine Learning (ML) specialists, and other supporting roles such as QA and product managers. Jane’s primary focus is on providing professional and experienced English-speaking AI developers to companies in the USA, Canada, and the UK.
Jane’s journey with AI began during her time at Duke University, where she pursued her studies in computer science. Her passion for AI grew exponentially as she delved into the intricacies of the subject. Over the years, she honed her skills and gained invaluable experience working with renowned companies such as Activision and the NSA. These experiences allowed her to master the art of integrating existing systems with AI APIs, solidifying her reputation as a versatile and resourceful AI professional.
Currently residing in the vibrant city of Los Angeles, Jane finds solace in her role as an author and developer. Outside of her professional pursuits, she cherishes the time spent with her two daughters, exploring the beautiful hills surrounding the city. Jane’s dedication to the advancement of AI technology, combined with her wealth of knowledge and experience, makes her an invaluable asset to the “Hire AI Developer” team and a trusted resource for readers seeking insights into the world of AI.