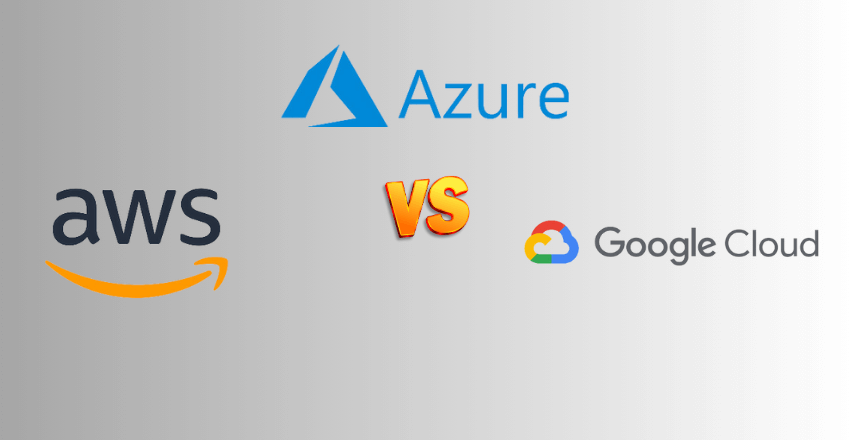
Azure vs AWS vs Google Cloud: the battle of cloud services intensifies.
Are you interested in developing AI-enabled systems but don’t know where to start? Look no further than the cloud. Choosing the right AI development platform is key to taking your project from concept to reality. With Azure, AWS, and Google Cloud as the top players in the market, it can be challenging to determine which platform is the best fit for your needs.
Cloud Computing Solutions
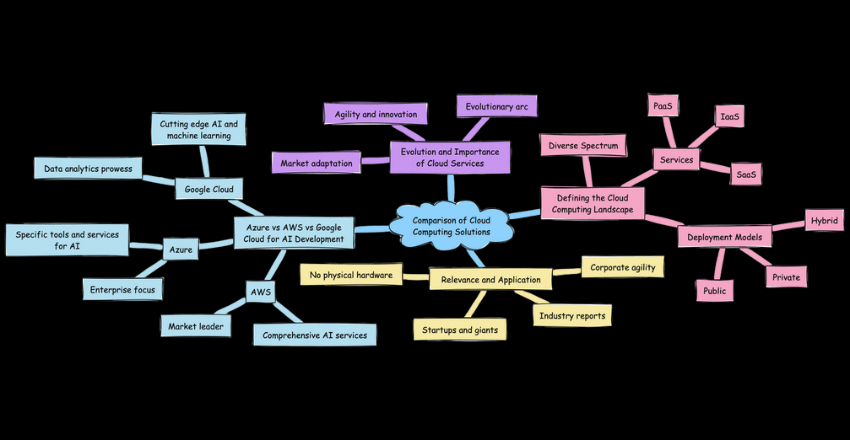
Cloud computing has undoubtedly become a cornerstone of innovation and efficiency in the contemporary digital landscape. As I delve into the intricacies of cloud computing comparison, one cannot overlook its transformative journey from a niche concept to a ubiquitous necessity in business operations.
Today, companies that leverage comprehensive cloud computing solutions gain significant competitive advantages, underscoring a paradigm shift in the way we perceive and utilize computational resources.
Evolution and Importance of Cloud Services
The evolutionary arc of cloud services is a testament to technological advancement and market adaptation. Let’s trace back to when the idea of network-based computing first began to materialize; it was a time when such an innovation promised greater agility for businesses.
Fast forward to the present, and this promise has been not just fulfilled but surpassed. By conducting a thorough cloud services comparison, we notice the exponential growth reflected in the pervasive adoption across industries—each vying for the benefits of scalability, cost-efficiency, and collaborative potency that these services provide.
Defining the Cloud Computing Landscape
In discussing the vast terrain of the cloud platform comparison, it becomes imperative to define the core services that constitute this burgeoning domain. The fundamental services encapsulate Infrastructure as a Service (IaaS), Platform as a Service (PaaS), and Software as a Service (SaaS), each offering varying degrees of control, flexibility, and management.
Meanwhile, the deployment models are delineated into public, private, and hybrid clouds, each presenting a unique set of features tailored to specific business requirements. This segmentation reaffirms the multifaceted nature of cloud computing, positioning it as a diverse spectrum of cloud computing solutions tailored to meet a wide array of operational demands.
Employing data from authoritative industry reports, we illuminate the staggering relevance and application of cloud services in today’s economy. From startups to giants, the reliance on cloud infrastructure is undeniable.
It has fueled innovation and enabled businesses to operate without the traditional confines of physical hardware investments—heralding a new era of corporate agility and dynamic market presence.

Azure vs AWS vs Google Cloud: A Comparative Analysis
Their fierce rivalry in market shares and technological advancements demands a meticulous comparison to understand their respective statures in the cloud provider arena. I’ve sourced recent market analysis to construct a factual and unbiased view of these entities.
Each of these cloud giants showcases a distinct portfolio of services encompassing Infrastructure as a Service (IaaS), Platform as a Service (PaaS), and Software as a Service (SaaS). However, their approach to delivering these services often diverges to cater to various segments and verticals.
For instance, Azure has carved out a niche in hybrid cloud solutions, which has resonated well with large enterprises, while AWS leads in comprehensive offerings, and Google Cloud excels with high data analytics and machine learning capabilities.
Scalability is another critical factor in the azure vs aws comparison, with both Microsoft Azure and AWS offering substantial scalability options for growing businesses. Google Cloud is slightly behind but is catching up rapidly, as evidenced by their recent expansions and enhancements in scalability features.
Similarly, when we talk about global reach, AWS has the most extensive infrastructure with the largest number of data centers worldwide, but Azure is close on its heels, leveraging Microsoft’s long-established global presence.
When we mention microsoft azure vs amazon web services vs google cloud platform, it’s essential to bring to light the developer ecosystem that each cloud provider nurtures. AWS shines with its broad scope of tools and community support, whereas Azure benefits from its seamless integration with other Microsoft products, which is a significant draw for developers versed in Microsoft’s ecosystem.
Meanwhile, Google Cloud is known for its deep investment in open source technologies and strong affinity with developers who prioritize modern development stacks.
To solidify our cloud provider comparison, let’s not overlook enterprise adoption levels which speak volumes about a provider’s reliability and industry reputation. AWS boasts a star-studded client list, emphasizing its extensive adoption across many industries.
Azure, with its compliance coverage, garners trust among businesses that hinge on rigorous regulatory requirements. Google Cloud, while having fewer enterprise customers compared to AWS and Azure, is rapidly gaining traction among new startups and data-centric companies.
Below is a comparative table that encapsulates key areas where these cloud service providers stand toe-to-toe:
Criteria | Microsoft Azure | Amazon Web Services | Google Cloud Platform |
---|---|---|---|
Market Share | Second Largest | Largest | Third Largest |
Service Offerings | Hybrid Cloud Focus | Most Comprehensive | Analytics & ML Focused |
Scalability | Extensive Options | Highly Scalable | Improving Rapidly |
Global Reach | Expansive | Most Extensive | Growing Presence |
Developer Ecosystem | Microsoft Integration | Vast Tooling | Open Source Support |
Enterprise Adoption | High in Regulated Industries | Widely Adopted | Gaining in Startups & Data-centric Companies |
This accumulated evidence underscores the dynamic nature of the cloud market and no single provider emerges as a universal front-runner. Each has carved out a space where it excels, pointing to the idea that the best choice often lies in aligning a business’s unique needs with the strengths of these platforms.
Navigating through the azure vs aws vs google cloud labyrinth, businesses must weigh these critical aspects to forge a path toward a scalable and innovative cloud ecosystem.
Breaking Down the Features
As we shift our focus to the crux of cloud service capabilities, a comparative approach unveils the technical nuances between Azure, AWS, and Google Cloud.
Computing Power and Storage Capabilities
In assessing cloud computing performance, the combatants, Azure, Google Cloud, and AWS, deploy an arsenal of solutions. Azure’s Blob Storage sets a standard in unstructured data storage, whereas AWS’s EC2 offers versatile computational provision with scalable storage through S3.
On the other side, Google Cloud’s Compute Engine furnishes flexible and efficient VMs with Persistent Disk, known for high-performance block storage. The cloud service provider comparison of these assets underlines the uniqueness and strengths each brings to the storage battleground, vital for informed decisions.
Networking and Security Features in the Public Cloud Providers
Delving into the heart of cloud service pricing and the implicit value, networking, and security features draw high attention. The core infrastructure of Azure, AWS, and Google Cloud exemplifies the focus on robust networks with their Virtual Network, VPC, and Cloud Load Balancing provisions respectively.
Built for protecting and interconnecting the myriad of cloud services, these features play a pivotal role in our cloud computing comparison—highlighting each provider’s commitment to safeguarding data while ensuring seamless connectivity.
Unique Offerings: AI, ML, and Analytics Tools
The dynamic and swiftly evolving landscape of cloud-based AI and analytics tools showcases a sharply competitive edge. Embarking on a comparative journey, Microsoft Azure’s Machine Learning Studio, AWS’s SageMaker, and Google Cloud’s AI platform each deliver powerful, accessible, and integrated solutions dictating the future of machine intelligence and data examination.
It’s this segment where the azure vs google cloud performance battle heats up, with each seeking dominance through innovation and specialized services that could revolutionize industries.
Focusing on Costs: Google Cloud vs AWS Pricing and Azure’s Price Structure
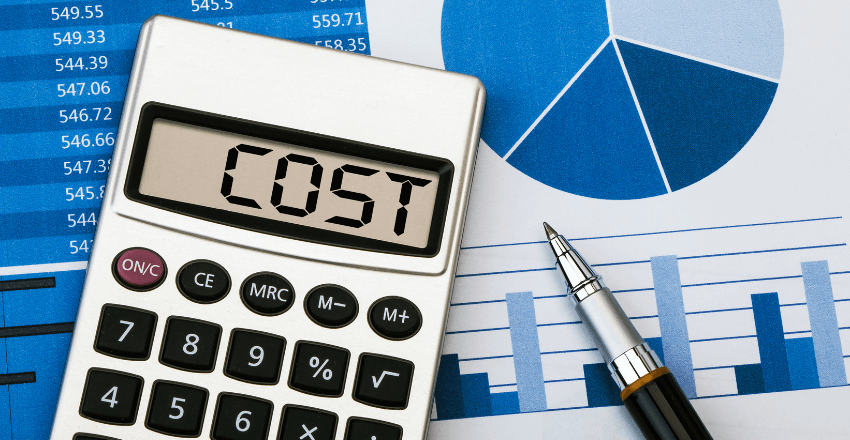
When it comes to selecting the best cloud provider, the financial implications often weigh as heavily as technical capabilities. I’ll now shed light on the distinctly varied pricing strategies of Google Cloud, AWS, and Azure, providing insight into each platform’s methodology for cloud service pricing.
In addition to subscription models, we’ll delve into the nuances of discounts, reserve instances, and commitments to help you comprehend the total cost of ownership in cloud platforms.
This consideration is essential, as pricing can significantly impact your overall cloud strategy and bottom-line outcomes.
Examining the Pricing Models of Top Cloud Vendors
Each cloud vendor has crafted its pricing model to cater to a range of customer needs. Google Cloud’s pricing is acknowledged for its customer-friendly approach, with pay-as-you-go flexibility and sustained use discounts that don’t require a long-term commitment.
AWS offers a similar on-demand structure but stands out with its Reserved Instances and Savings Plans, enticing businesses with substantial discounts for pre-committing to usage. Microsoft’s Azure, on the other hand, fosters a blend of pay-as-you-go solutions with possible cost reductions via their Azure Reserved Virtual Machine Instances. Understanding these models is key to realizing potential savings.
Understanding Total Cost of Ownership in Cloud Platforms
The total cost of ownership extends beyond just the advertised pricing; it encapsulates various factors that affect your expenses in the long term. Indirect costs, such as network data transfer fees, storage transactions, and operational expenses must be accounted for. My analysis delves into how each cloud provider calculates these costs, shedding light on the often-overlooked aspects of cloud economics.
By dissecting these elements, businesses can envision a more complete financial picture and plan their investments accordingly.
How to Estimate Your Cloud Budget Accurately
Estimating a cloud budget demands a meticulous approach where I recommend considering not only direct compute and storage needs but also potential growth, data egress fees, and the scaling nature of your applications.
AWS’s calculator, Google Cloud’s pricing calculator, and Azure’s pricing calculator offer starting points for forecasting expenses tailored to anticipated usage patterns.
Final Thoughts
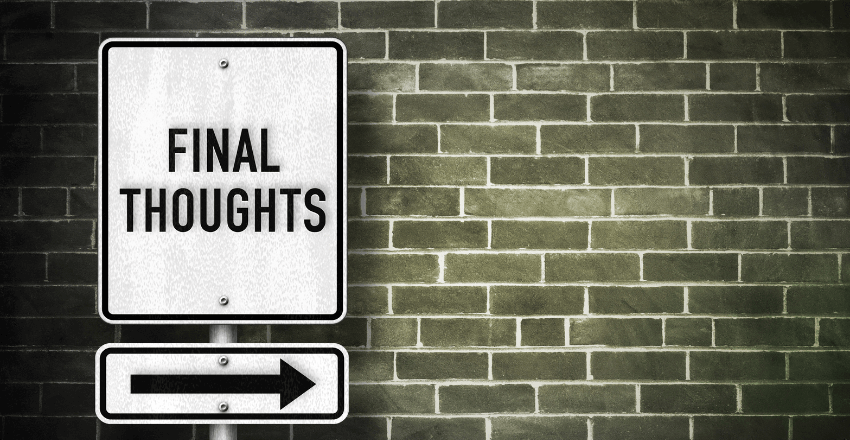
My final words linger on the premise that the cloud landscape is perpetually evolving, with each provider relentlessly enhancing their technologies to capture the essence of future-ready businesses. There is no universal one-size-fits-all solution; the approach must be as agile and dynamic as the cloud itself.
Therefore, I urge companies to remain astutely aware of this maturing ecosystem, to glean insights articulated through this analysis, in order to forge a partnership with a cloud provider that not only meets today’s expectations but is also equipped to scale the heights of tomorrow’s potentials.
About Hire AI Developer
Hire AI Developer is a leading provider of AI development services, with a team of experienced developers dedicated to delivering high-quality AI solutions to clients across industries. Our mission is to harness the power of AI to drive innovation and create value for our clients, helping them stay ahead of the competition in a rapidly changing digital landscape.
We specialize in developing AI-enabled systems that can solve complex business problems, leveraging the latest technologies and methodologies to deliver results that exceed expectations. Whether you’re looking to build a customized AI solution from scratch or optimize an existing system, our team has the expertise and experience to help you succeed.
At Hire AI Developer, we recognize the importance of staying up-to-date with the latest AI development platforms and solutions in the cloud. That’s why we’re committed to continuous learning and development, ensuring that our team has the knowledge and skills necessary to deliver cutting-edge solutions that drive results.
If you’re looking for a trusted partner in AI development, look no further than Hire AI Developer. Contact us today to learn how we can help you achieve your AI development goals.
External Resources
https://cloud.google.com/?hl=en
https://azure.microsoft.com/en-us
FAQ

1. How do I start a virtual machine on Azure, AWS, and Google Cloud?
Azure:
from azure.identity import DefaultAzureCredential
from azure.mgmt.compute import ComputeManagementClient
credential = DefaultAzureCredential()
subscription_id = 'your-subscription-id'
compute_client = ComputeManagementClient(credential, subscription_id)
vm_start = compute_client.virtual_machines.begin_start(
"your-resource-group", "your-vm-name")
vm_start.wait()
This Python script uses Azure SDK to authenticate and start a VM in your Azure account.
AWS:
import boto3
ec2 = boto3.client('ec2', region_name='your-region')
response = ec2.start_instances(
InstanceIds=[
'your-instance-id',
]
)
Boto3, the AWS SDK for Python, is used here to start an EC2 instance.
Google Cloud:
from google.oauth2 import service_account
from googleapiclient import discovery
credentials = service_account.Credentials.from_service_account_file(
'your-service-account-file.json')
service = discovery.build('compute', 'v1', credentials=credentials)
project = 'your-project-id'
zone = 'your-zone'
instance = 'your-instance-name'
request = service.instances().start(project=project, zone=zone, instance=instance)
response = request.execute()
This snippet uses Google Cloud Python Client Library to authenticate and start a Compute Engine instance.
2. How can I create a storage bucket in Azure, AWS, and Google Cloud?
Azure (Blob Storage):
from azure.storage.blob import BlobServiceClient, PublicAccess
service_client = BlobServiceClient.from_connection_string('your-connection-string')
container_client = service_client.create_container("your-container-name",
public_access=PublicAccess.Container)
Creates a container in Azure Blob Storage, which is analogous to a bucket.
AWS (S3):
import boto3
s3 = boto3.client('s3')
s3.create_bucket(Bucket='your-bucket-name')
Uses Boto3 to create a new S3 bucket.
Google Cloud (Storage):
from google.cloud import storage
client = storage.Client()
bucket = client.create_bucket('your-bucket-name')
This code uses Google Cloud Storage Client Library to create a new storage bucket.
3. How do I deploy a containerized application on Azure, AWS, and Google Cloud?
Azure (Azure Container Instances):
from azure.identity import DefaultAzureCredential
from azure.mgmt.containerinstance import ContainerInstanceManagementClient
from azure.mgmt.containerinstance.models import ContainerGroup, Container, ContainerPort, ImageRegistryCredential
credential = DefaultAzureCredential()
client = ContainerInstanceManagementClient(credential, 'your-subscription-id')
container_group = client.container_groups.begin_create_or_update(
"your-resource-group",
"your-container-group-name",
ContainerGroup(
location="your-location",
containers=[
Container(
name="your-container-name",
image="your-container-image",
resources={
"requests": {
"cpu": 1.0,
"memory_in_gb": 1.5
}
},
ports=[ContainerPort(port=80)]
)
],
os_type="Linux"
)
)
Deploys a containerized application using Azure Container Instances.
AWS (ECS with Fargate):
import boto3
ecs = boto3.client('ecs')
response = ecs.create_service(
cluster='your-cluster-name',
serviceName='your-service-name',
taskDefinition='your-task-definition',
launchType='FARGATE',
desiredCount=1,
networkConfiguration={
'awsvpcConfiguration': {
'subnets': ['your-subnet-ids'],
'assignPublicIp': 'ENABLED'
}
}
)
Sets up a container service using AWS Fargate on ECS.
Google Cloud (Cloud Run):
from google.cloud import run_v2
from google.cloud.run_v2 import TrafficTarget
service_client = run_v2.ServicesClient()
service = run_v2.Service(
name='projects/your-project-id/locations/your-location/services/your-service-name',
template=run_v2.RevisionTemplate(
containers=[run_v2.Container(
image='your-container-image'
)]
),
traffic=[TrafficTarget(
type_='SERVING',
percent=100
)]
)
operation = service_client.create_service(
parent='projects/your-project-id/locations/your-location',
service=service,
service_id='your-service-id'
)
result = operation.result()
Deploys a container to Google Cloud Run, a managed platform for containerized applications.
4. How can I set up a managed database on Azure, AWS, and Google Cloud?
Azure (Azure SQL Database):
from azure.identity import DefaultAzureCredential
from azure.mgmt.sql import SqlManagementClient
from azure.mgmt.sql.models import Server, Database
credential = DefaultAzureCredential()
sql_client = SqlManagementClient(credential, 'your-subscription-id')
# Create SQL Server
server = sql_client.servers.begin_create_or_update(
"your-resource-group",
"your-sql-server-name",
Server(
location="your-location",
administrator_login="your-admin-login",
administrator_login_password="your-admin-password"
)
).result()
# Create Database on the Server
database = sql_client.databases.begin_create_or_update(
"your-resource-group",
"your-sql-server-name",
"your-database-name",
Database(location="your-location")
).result()
Creates a SQL server and database in Azure.
AWS (RDS):
import boto3
rds = boto3.client('rds')
db_instance = rds.create_db_instance(
DBInstanceIdentifier='your-db-instance-identifier',
AllocatedStorage=20,
DBInstanceClass='db.t2.micro',
Engine='mysql',
MasterUsername='your-username',
MasterUserPassword='your-password'
)
Sets up a MySQL instance on Amazon RDS, AWS’s managed relational database service.
Google Cloud (Cloud SQL):
from google.cloud import sql_v1
sql_admin_client = sql_v1.SqlInstancesServiceClient()
instance = sql_v1.DatabaseInstance(
name='your-instance-name',
settings=sql_v1.Settings(
tier='db-f1-micro',
ip_configuration=sql_v1.IpConfiguration(
ipv4_enabled=True
)
),
database_version=sql_v1.SqlDatabaseVersion.MYSQL_5_7,
region='your-region'
)
response = sql_admin_client.insert(
project='your-project-id',
instance=instance
)
Launches a MySQL database instance using Google Cloud SQL, a fully managed database service.
5. How do I manage authentication across Azure, AWS, and Google Cloud?
Azure (Azure Active Directory):
from azure.identity import DefaultAzureCredential
credential = DefaultAzureCredential()
# Use credential to authenticate against Azure services
Leverages Azure Active Directory (AAD) for managing identities and authentication.
AWS (IAM Roles):
import boto3
session = boto3.Session()
sts = session.client('sts')
assumed_role = sts.assume_role(
RoleArn='your-role-arn',
RoleSessionName='your-session-name'
)
# Use the assumed role credentials for authentication
Utilizes AWS Identity and Access Management (IAM) roles for secure access to AWS services.
Google Cloud (Service Accounts):
from google.oauth2 import service_account
credentials = service_account.Credentials.from_service_account_file(
'your-service-account-file.json')
# Use credentials to authenticate against Google Cloud services
Employs Google Cloud service accounts for secure, role-based access to resources.

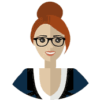
Jane Watson is a seasoned expert in AI development and a prominent author for the “Hire AI Developer” blog. With over a decade of experience in the field, Jane has established herself as a leading authority in AI app and website development, as well as AI backend integrations. Her expertise extends to managing dedicated development teams, including AI developers, Machine Learning (ML) specialists, and other supporting roles such as QA and product managers. Jane’s primary focus is on providing professional and experienced English-speaking AI developers to companies in the USA, Canada, and the UK.
Jane’s journey with AI began during her time at Duke University, where she pursued her studies in computer science. Her passion for AI grew exponentially as she delved into the intricacies of the subject. Over the years, she honed her skills and gained invaluable experience working with renowned companies such as Activision and the NSA. These experiences allowed her to master the art of integrating existing systems with AI APIs, solidifying her reputation as a versatile and resourceful AI professional.
Currently residing in the vibrant city of Los Angeles, Jane finds solace in her role as an author and developer. Outside of her professional pursuits, she cherishes the time spent with her two daughters, exploring the beautiful hills surrounding the city. Jane’s dedication to the advancement of AI technology, combined with her wealth of knowledge and experience, makes her an invaluable asset to the “Hire AI Developer” team and a trusted resource for readers seeking insights into the world of AI.